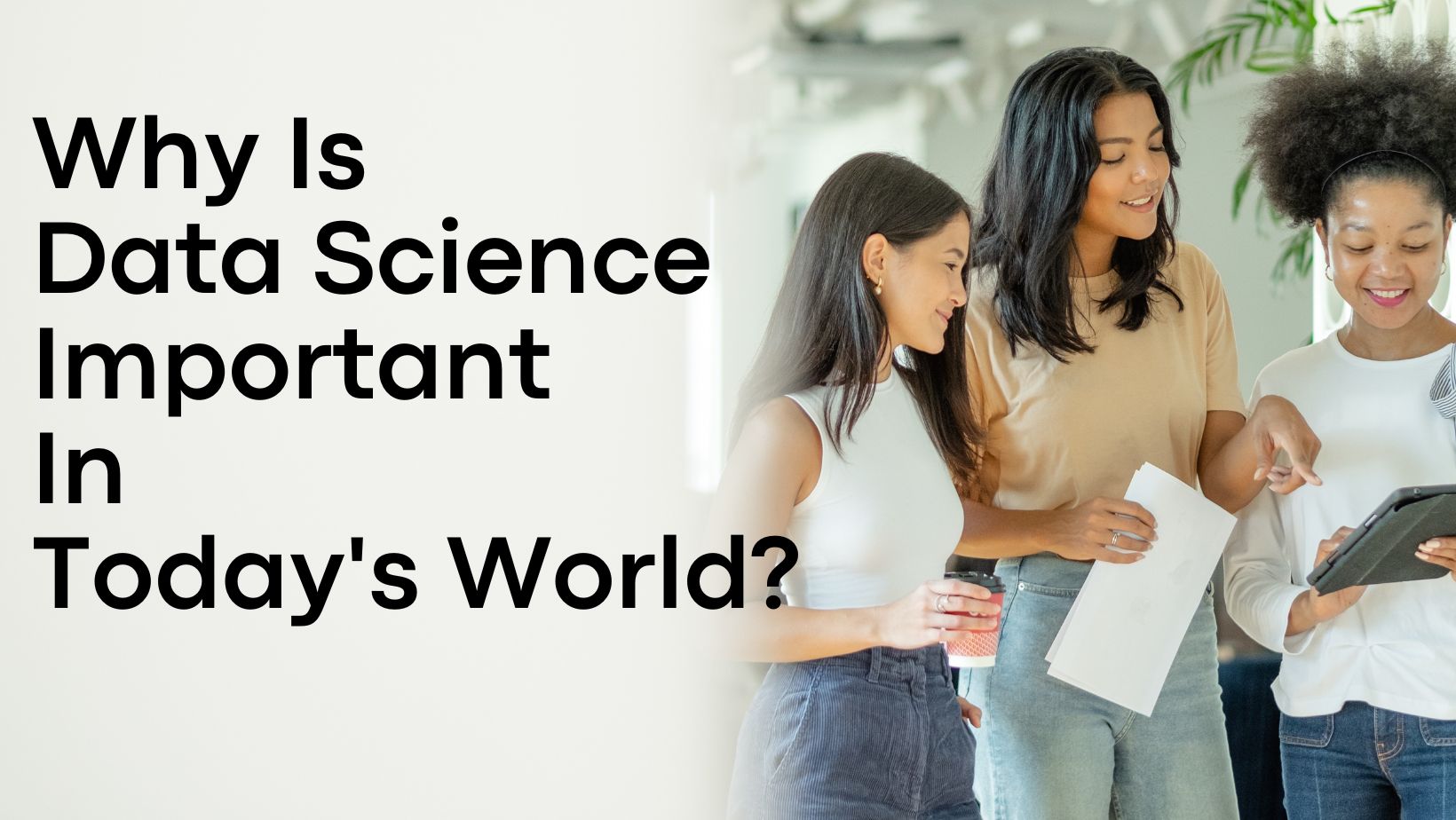
Introduction
Data science has become a cornerstone of the modern digital age, transforming the way we analyze and interpret information. From businesses making data-driven decisions to healthcare improving patient outcomes, data science impacts almost every sector. In today's world, where data is generated at an unprecedented rate, understanding the significance of data science is crucial.
What Is Data Science?
Data science is an interdisciplinary field that combines statistics, computer science, and domain expertise to extract insights from structured and unstructured data. It involves processes like data collection, cleaning, analysis, and visualization to solve complex problems and predict future trends.
Why Has Data Science Gained Popularity?
- Explosion of Data: The digital age has led to an exponential increase in the volume of data generated every day. From social media posts to e-commerce transactions, data is being created at a staggering pace.
- Advancements in Technology: With the advent of cloud computing, artificial intelligence (AI), and machine learning (ML), data science has become more accessible and powerful.
- Business Competitiveness: Companies that leverage data science gain a competitive edge, making them more efficient and capable of meeting customer demands.
The Role of Data Science in Various Sectors
Data Science in Business
- Enhancing Decision-Making: Businesses can use data science to make informed decisions by analyzing market trends, customer preferences, and sales data. This helps in strategic planning and forecasting.
- Personalized Customer Experiences: By analyzing customer behavior, businesses can tailor their products and services to individual needs, improving customer satisfaction and retention.
- Operational Efficiency: Data science aids in optimizing processes, reducing costs, and improving productivity by identifying inefficiencies in business operations.
Data Science in Healthcare
- Predictive Analytics: Healthcare providers use data science to predict disease outbreaks, patient admissions, and treatment outcomes, which can significantly improve patient care.
- Personalized Medicine: Data analysis allows doctors to create personalized treatment plans based on a patient’s genetic makeup and medical history, leading to better outcomes.
- Medical Image Analysis: Machine learning algorithms are used to analyze medical images like X-rays, MRIs, and CT scans, helping in early diagnosis and treatment.
Data Science in Finance
- Risk Management: Financial institutions use data science to assess risks, detect fraudulent activities, and determine creditworthiness.
- Algorithmic Trading: Data-driven models are used in stock market trading to predict market movements and automate trading processes.
- Customer Insights: By analyzing transaction data, banks can understand customer behavior, leading to the creation of personalized financial products.
Data Science in E-commerce
- Recommendation Systems: Data science powers recommendation engines used by e-commerce platforms, which suggest products to customers based on their browsing history and preferences.
- Inventory Management: Data analytics helps e-commerce companies manage their inventory efficiently by predicting demand and avoiding stockouts.
- Customer Sentiment Analysis: Analyzing customer reviews and feedback through natural language processing (NLP) helps businesses understand customer sentiments and improve their products.
Data Science in Transportation
- Optimizing Routes: Data science is used to optimize delivery routes, reducing fuel consumption and ensuring timely deliveries in logistics.
- Predicting Traffic Patterns: Data analysis helps in predicting traffic congestion and developing solutions to manage it, leading to better traffic flow in urban areas.
- Self-Driving Cars: Autonomous vehicles rely on data science for object detection, route planning, and real-time decision-making.
Key Components of Data Science
- Data Collection: Gathering data from various sources like databases, APIs, and web scraping.
- Data Cleaning: Cleaning the data to remove inconsistencies, duplicates, and missing values.
- Data Analysis: Using statistical methods and algorithms to analyze the data and identify patterns.
- Data Visualization: Creating visual representations like graphs and charts to communicate findings effectively.
- Modeling and Prediction: Building machine learning models to make predictions or classify data points.
The Growing Need for Data Science Training
As data science continues to gain prominence, there is a rising demand for skilled professionals in this field. This has led to the proliferation of specialized training programs across the country. Whether you're just starting out or looking to enhance your skills, a data science training course in Noida, Delhi, Meerut, Chandigarh, Pune, and other cities located in India can provide the knowledge and hands-on experience necessary to succeed. Such courses are designed to equip learners with the latest tools and techniques, enabling them to tackle real-world data challenges effectively.
Impact of Data Science on Society
- Improving Public Policy: Governments use data science to improve policy-making, predict social trends, and make data-driven decisions for public welfare.
- Advancing Education: Educational institutions utilize data science to develop personalized learning experiences and track student performance.
- Sustainable Development: Data science helps in analyzing environmental data to track climate changes, reduce waste, and promote sustainability.
Challenges in Data Science
- Data Privacy Concerns: As data science relies heavily on user data, ensuring privacy and security is a major challenge.
- Data Quality: The accuracy of data is crucial for generating reliable insights. Inaccurate or incomplete data can lead to misleading conclusions.
- Skill Gap: The demand for skilled data scientists far exceeds the supply, creating a gap that companies struggle to fill.
- Ethical Issues: Using data science responsibly is important to avoid biases and ensure that models do not perpetuate discrimination.
Future Trends in Data Science
- Artificial Intelligence Integration: AI and ML will become even more integrated into data science processes, making predictions more accurate.
- Real-time Analytics: The focus will shift towards analyzing data in real-time, providing immediate insights and actionable data.
- Augmented Analytics: Tools that use AI to enhance data analysis, enabling even non-technical users to gain insights, will become more common.
- Edge Computing: With the rise of IoT, data processing will shift closer to the data source, allowing for faster and more efficient analysis.
Conclusion
Data science is not just a trend but a necessity in today's data-driven world. Its ability to turn raw data into actionable insights has made it indispensable across various industries. As data continues to grow exponentially, so does the potential of data science to solve real-world problems, drive innovation, and shape the future. Embracing data science is crucial for individuals and businesses to stay competitive and relevant in this evolving landscape.
We do not claim ownership of any content, links or images featured on this post unless explicitly stated. If you believe any content or images infringes on your copyright, please contact us immediately for removal ([email protected]). Please note that content published under our account may be sponsored or contributed by guest authors. We assume no responsibility for the accuracy or originality of such content. We hold no responsibilty of content and images published as ours is a publishers platform. Mail us for any query and we will remove that content/image immediately.